Manual quality control methods limit the efficiency and effectiveness of modern manufacturing processes.
For one, manual inspection is too inconsistent. Due to factors like fatigue, human inspectors are prone to variability in their defect detection rates, which can range between 60 and 90 percent.
Additionally, manual inspection is also slow. Manual processes often cannot keep up with fast-moving assembly lines, resulting in bottlenecks.
Another challenge is the high labor costs associated with employing qualified quality control (QC) inspectors. In the United States, the average salary for a QC inspector is more than $89,000 per year. These costs tend to increase as production scales.
Finally, manual quality control methods often lack the capability to provide real-time data. This limitation can lead to prolonged periods before defects are detected, which can be costly in terms of both time and resources.
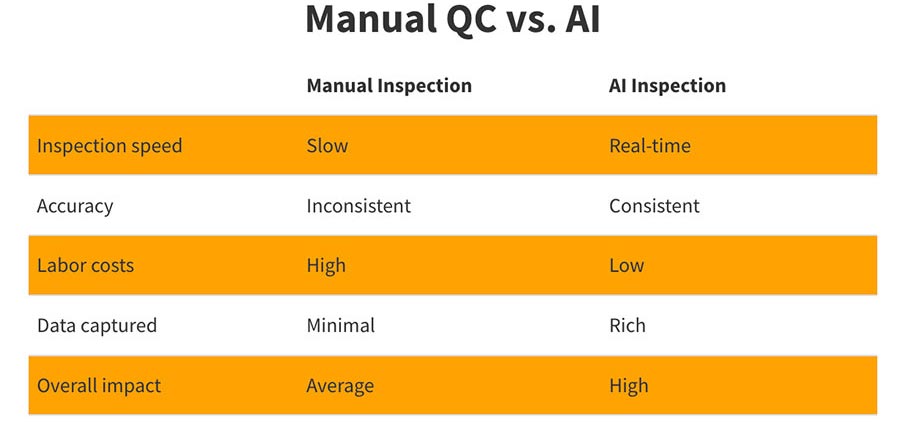
Source: EasyODM.tech
Advantages of AI
In contrast, quality control technology driven by artificial intelligence offers numerous advantages that address these challenges.
Automated optical inspection, powered by AI algorithms, can reliably detect defects and quality issues with a level of attention and accuracy that human inspectors are unable to achieve consistently.
This technology provides real-time intelligence, enabling factories to immediately identify and rectify quality issues as they arise. As a result, AI-driven quality control not only reduces the time needed to detect and address problems, but also significantly improves the overall product quality.
For large manufacturers, implementing AI-driven quality control can lead to substantial cost reductions. By automating the inspection process and enhancing product quality, manufacturers can achieve more efficient workflows and reduce the expenses associated with manual quality control methods.
How Does AI Quality Inspection Work?
The AI-driven quality inspection is a multistage process designed to ensure precision and efficiency in product evaluation. Here’s an overview of how an AI system operates, from the arrival of products at the inspection area to the final decision-making and documentation stages:

AI-based inspection is a six-step process. Illustration courtesy EasyODM.tech
- Arrival at inspection area. The product is brought from the assembly line to the inspection area and positioned for assessment.
- Image capture. High-quality cameras capture detailed images of the product, with lighting and positioning adjusted based on inspection requirements.
- Image preprocessing. The system determines whether to use gray-scale or color images, performing image transformations for optimal analysis.
- Defect detection. A custom convolutional neural network (CNN) architecture analyzes the images. The network is trained to identify features of defective and non-defective products.
- Decision stage. The operator reviews the AI-generated results and decides whether to accept or reject the product based on the inspection findings.
- Documentation. Inspection results are recorded in the shop floor quality improvement software and logged systematically.
Best Practices in AI Inspection
When deploying AI-driven inspection systems, it’s crucial to address a range of challenges while adhering to best practices for optimal results.
One of the primary challenges is ensuring the clarity and accuracy of images used for AI model predictions. High-quality images are essential, since they directly affect the reliability of the model. Another significant challenge is the gap in training data, especially the limited availability of defect samples, which are critical for accurate model training.
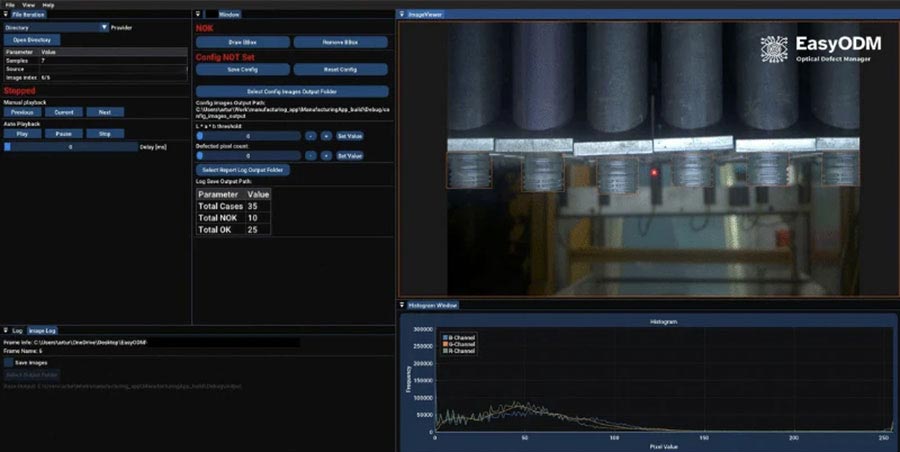
A custom convolutional neural network architecture analyzes the images. The network is trained to identify features of defective and non-defective products. Illustration courtesy EasyODM.tech
Maintaining model accuracy is also a key concern. This involves regular validation and tuning of AI models to enhance their classification capabilities. Additionally, there are hardware constraints to consider. Striking a balance between camera resolution, model complexity, and the capabilities of available hardware is essential.
Another aspect to manage is model drift, which involves updating AI models to adapt to changes in product materials or specifications.
To implement AI effectively, several best practices are recommended. Starting with small-scale pilot projects on a limited product range allows for a gradual and controlled introduction of AI systems. Simplifying and standardizing data collection is crucial for ensuring model accuracy.
It’s important to monitor model metrics closely. This includes tracking performance indicators and regularly updating models to ensure they remain effective. Rapid retraining of models for new defects or products is essential to keep the AI system agile and responsive.
Broadly deploying AI systems across various production stages can maximize their benefits. Integrating AI insights with IoT sensor data can further enhance the depth and utility of the analysis.
Lastly, ensuring smooth integration of AI findings into central quality databases is crucial for comprehensive and effective quality management. These practices help facilitate a smoother transition to AI-driven inspection systems, leading to higher efficiency and accuracy in manufacturing quality control.
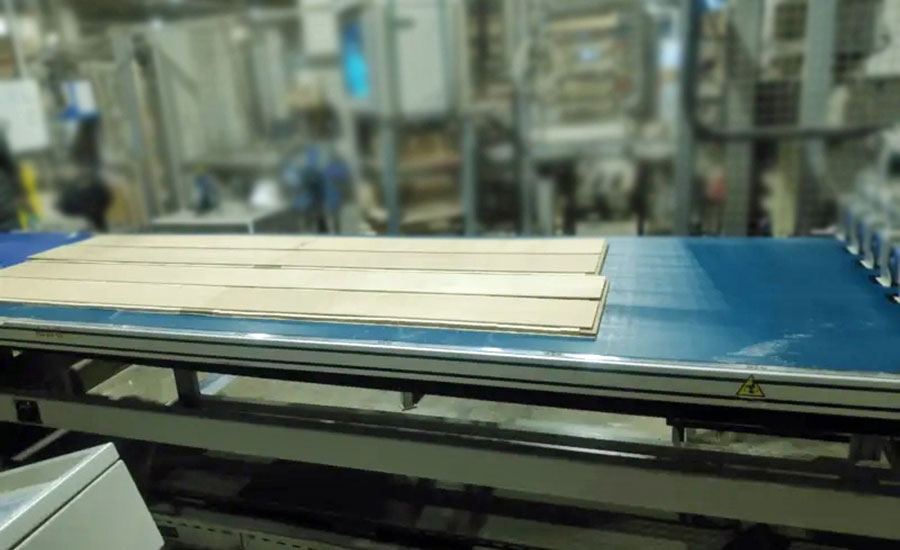
A manufacturer of hardwood flooring uses AI to find defects in boards. Illustration courtesy EasyODM.tech
Common Use Cases
AI-driven quality control is increasingly vital for ensuring product excellence and consistency. This technology has been implemented in a variety of ways to enhance the efficiency and accuracy of quality inspections.
One application of AI in quality inspection is surface defect detection. AI systems scan materials such as steel, wood, glass, plastic or textiles, identifying surface flaws that could compromise the quality of the product. Similarly, AI can be used for structural and dimensional checking, where it precisely measures product dimensions and flags any deviations from specified standards.
Another important use of AI is in assembly verification. This involves confirming the accuracy of assembled products against their bill-of-materials, ensuring that each component is correctly placed and present. AI is also used for inspecting labels, packaging and artwork, which are essential for brand image and regulatory compliance. AI ensures these are accurate and consistent.
Additionally, AI is adept at cosmetic flaw detection, identifying tiny blemishes and imperfections in products that might be missed by the human eye. In the realm of anomaly detection, AI flags products that deviate from established quality standards, helping maintain uniformity across production batches.
AI’s role in defect detection is particularly notable in intricate products like electronics and automotive parts. It identifies flaws with precision and speed. Moreover, AI assists in packaging inspection, ensuring its integrity and accuracy.
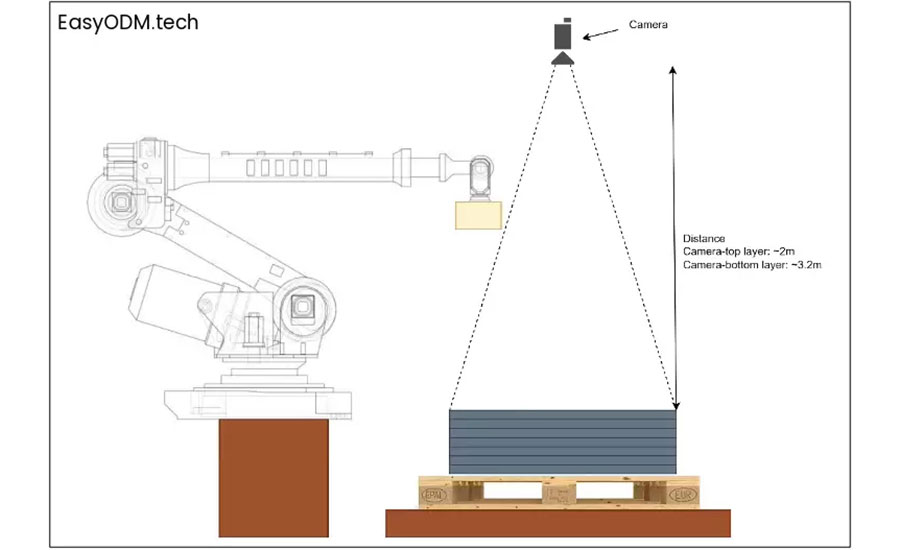
A furniture manufacturer uses an AI-based vision system to automate a palletizing operation. Illustration courtesy EasyODM.tech
Material sorting and product counting are other areas where AI can help. It sorts materials based on quality using visual inspection and automates counting for inventory management. In the assembly process, AI provides robotic guidance, assisting in the accurate placement of parts.
Lastly, AI is instrumental in safety compliance monitoring, ensuring adherence to safety standards and thus safeguarding both product quality and the work environment.
Real-World Use Case
A leading manufacturer of car seats faced significant challenges with its manual inspection process. The primary issue was the detection of wrinkle defects on the seats, a task that was time-consuming and prone to human error. This inefficiency led to high defect rates, compromised quality, and increased costs.
To address these challenges, the manufacturer deployed EasyODM’s AI-driven inspection technology. This system employed machine vision and AI algorithms specifically designed to analyze seat materials and detect defects.
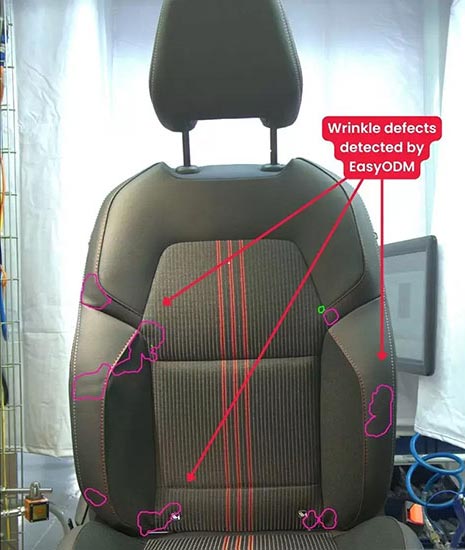
A manufacturer of car seats uses AI to detect wrinkles in upholstery. Photo courtesy EasyODM.tech
The system was able to identify defects with a 99 percent accuracy. A key advantage of EasyODM’s system was that it could be integrated into the existing production line without any need for modification, allowing for a seamless transition to the new inspection method.
Once the AI-powered machine vision inspection identified wrinkles, the seats were automatically transferred to a six-axis robot equipped with an iron. This robot was specially designed to smooth out the wrinkles, ensuring that each seat met the highest quality standards. This integration of AI inspection with robotic correction proved to be highly effective.
The results of implementing EasyODM’s solution were significant. The manufacturer saw a 30 percent reduction in defect rates. Moreover, the inspection time was reduced from 1 minute per seat in the manual process to just 2.2 seconds per seat using the AI system. In terms of cost savings, the company experienced a 30-fold reduction in costs compared with manual inspection.
The return on investment for the AI-driven quality control system was impressive. The manufacturer achieved full ROI in less than two years.
The future of manufacturing is here, and it’s being driven by AI.
ASSEMBLY ONLINE
For more information on AI and machine vision, read these articles:
AI Augments Machine Vision
Deep Learning Machine Vision
AI-Based Visual Inspection for Spark Plug Manufacturing